Bagging¶
Bagging can be used for regression or classification, though we will demonstrate a regression bagging model here. Since this model is based on decision tree regressors, we’ll first import our regression tree construction from the previous chapter. We’ll also import numpy and the visualization packages.
## Import decision trees
import import_ipynb
import regression_tree as rt;
## Import numpy and visualization packages
import numpy as np
import matplotlib.pyplot as plt
import seaborn as sns
from sklearn import datasets
We will build our bagging model on the tips dataset from scikit-learn
. The hidden code cell below loads that data and does a train-test split.
## Load data
tips = sns.load_dataset('tips')
X = np.array(tips.drop(columns = 'tip'))
y = np.array(tips['tip'])
## Train-test split
np.random.seed(1)
test_frac = 0.25
test_size = int(len(y)*test_frac)
test_idxs = np.random.choice(np.arange(len(y)), test_size, replace = False)
X_train = np.delete(X, test_idxs, 0)
y_train = np.delete(y, test_idxs, 0)
X_test = X[test_idxs]
y_test = y[test_idxs]
Now we can get right into the bagging class. To fit the Bagger
object, we provide training data, the number of bootstraps (B
), and size regulation parameters for the decision trees. The object then takes B
bootstraps of the data, each time fitting a decision tree regressor. To form predictions with the Bagger
object, we simply run test observations through each bootstrapped tree and average the fitted values.
class Bagger:
def fit(self, X_train, y_train, B, max_depth = 100, min_size = 2, seed = None):
self.X_train = X_train
self.N, self.D = X_train.shape
self.y_train = y_train
self.B = B
self.seed = seed
self.trees = []
np.random.seed(seed)
for b in range(self.B):
sample = np.random.choice(np.arange(self.N), size = self.N, replace = True)
X_train_b = X_train[sample]
y_train_b = y_train[sample]
tree = rt.DecisionTreeRegressor()
tree.fit(X_train_b, y_train_b, max_depth = max_depth, min_size = min_size)
self.trees.append(tree)
def predict(self, X_test):
y_test_hats = np.empty((len(self.trees), len(X_test)))
for i, tree in enumerate(self.trees):
y_test_hats[i] = tree.predict(X_test)
return y_test_hats.mean(0)
We can now fit the bagging model and display the observed versus fitted values.
## Build model
bagger = Bagger()
bagger.fit(X_train, y_train, B = 30, max_depth = 20, min_size = 5, seed = 123)
y_test_hat = bagger.predict(X_test)
## Plot
fig, ax = plt.subplots(figsize = (7, 5))
sns.scatterplot(y_test, y_test_hat)
ax.set(xlabel = r'$y$', ylabel = r'$\hat{y}$', title = r'Observed vs. Fitted Values for Bagging')
sns.despine()
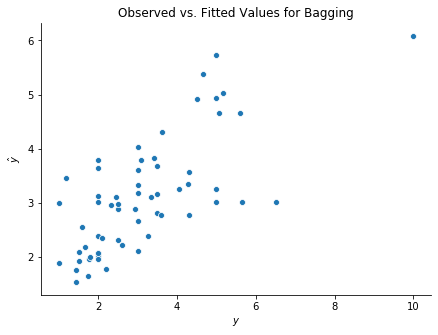