Random Forests¶
Fitting the random forest is essentially identical to fitting the bagging model with one exception: we tell the decision trees to consider only a subset of the predictors at each split. The code to do this is actually written in the decision tree construction section.
As in the bagging model, let’s import the regression tree construction and load the tips dataset. This is done in the hidden code cell below.
## Import decision trees
import import_ipynb
import regression_tree as rt;
## Import numpy and visualization packages
import numpy as np
import matplotlib.pyplot as plt
import seaborn as sns
from sklearn import datasets
## Load data
tips = sns.load_dataset('tips')
X = np.array(tips.drop(columns = 'tip'))
y = np.array(tips['tip'])
## Train-test split
np.random.seed(1)
test_frac = 0.25
test_size = int(len(y)*test_frac)
test_idxs = np.random.choice(np.arange(len(y)), test_size, replace = False)
X_train = np.delete(X, test_idxs, 0)
y_train = np.delete(y, test_idxs, 0)
X_test = X[test_idxs]
y_test = y[test_idxs]
We now build the RandomForest
class in the same way we built the Bagger
with one difference: we add the argument C
which controls the number of predictors the decision trees consider at each split.
class RandomForest:
def fit(self, X_train, y_train, B, C, max_depth = 100, min_size = 2, seed = None):
self.X_train = X_train
self.N, self.D = X_train.shape
self.y_train = y_train
self.B = B
self.C = C
self.seed = seed
self.trees = []
np.random.seed(seed)
for b in range(self.B):
sample = np.random.choice(np.arange(self.N), size = self.N, replace = True)
X_train_b = X_train[sample]
y_train_b = y_train[sample]
tree = rt.DecisionTreeRegressor()
tree.fit(X_train_b, y_train_b, max_depth = max_depth, min_size = min_size, C = C)
self.trees.append(tree)
def predict(self, X_test):
y_test_hats = np.empty((len(self.trees), len(X_test)))
for i, tree in enumerate(self.trees):
y_test_hats[i] = tree.predict(X_test)
return y_test_hats.mean(0)
We can now build a random forest and compare the observed with fitted values, as follows.
## Build model
rf = RandomForest()
rf.fit(X_train, y_train, B = 30, C = 4, max_depth = 20, min_size = 5, seed = 123)
y_test_hat = rf.predict(X_test)
## Plot
fig, ax = plt.subplots(figsize = (7, 5))
sns.scatterplot(y_test, y_test_hat)
ax.set(xlabel = r'$y$', ylabel = r'$\hat{y}$', title = r'Random Forest Observed vs. Fitted Values')
sns.despine()
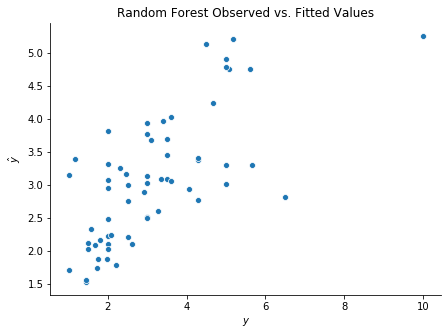